Data Scrub: SoReMo Fellows Learn the Difficulty of Finding Clean Data for Research
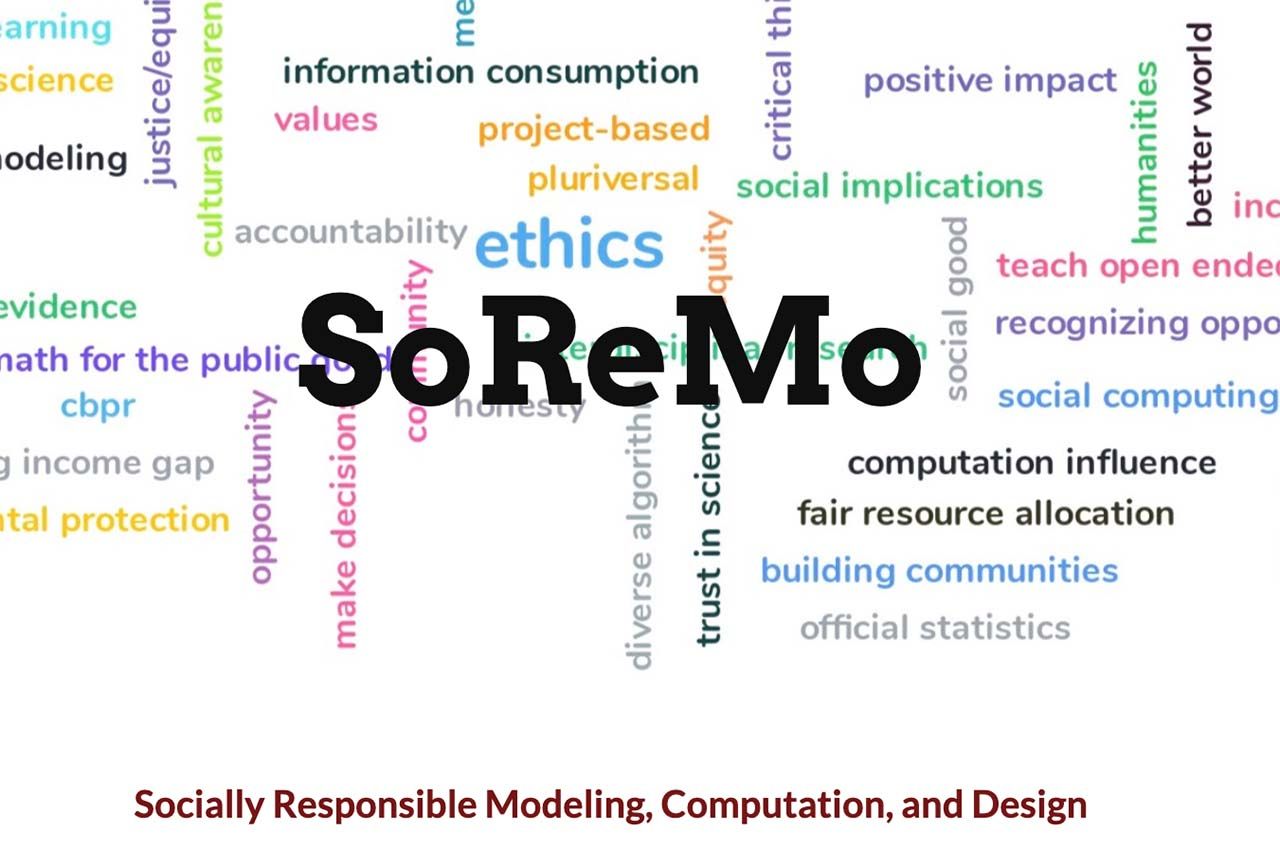
Finding good, clean data can be difficult while conducting research, and the fellows of the Socially Responsible Modeling, Computation, and Design (SoReMo) initiative at Illinois Institute of Technology learned that hard lesson during the fall 2021 semester.
Each fellow conducted their own semester-long research project and had their work published in the latest issue of SoReMo Journal. The SoReMo initiative empowers students to enact positive societal change within the Illinois Tech community, Chicago, and beyond by sponsoring them to conduct research projects that they are passionate about.
This university-wide initiative advocates for ethical, equitable approaches in computation, modeling, and design that contributes to the common good through research and education initiatives at Illinois Tech.
Sara Simon (Ph.D. DHUM Student) examined the challenges of collecting and reporting data as a new emergency threatens public health in her project “The Data-Driven Narratives of Epidemics: A History of Chicago’s Public Health Data Pipelines.”
Many jurisdictions have been collecting COVID-19 data at the local, state, and federal levels. Simon says each jurisdiction has its own way of reporting this data. Although the data might all be about the spread of COVID-19, each data set might be reporting on slightly different aspects of the disease, leading to classification discrepancies. Sometimes reports go to the wrong jurisdiction. Information also is hindered by outdated data systems that rely on paper and fax machines. Sometimes older data is reclassified and released with new data. Also, the demand for testing has overwhelmed lab capacities, which create data reporting backlogs.
“The data is not as precise as we might expect or want it to be, and as researchers that’s really frustrating. We want to get our hands on good clean data,” Simon says. “This doesn’t mean the data shouldn’t be used or trusted.”
Rather, Simon says researchers need to pay close attention to the footnotes that accompany data, which give more details as to when the information was collected and reported. Taking that information into account when using it will help researchers when they apply it to their models.
This imprecise data may have affected Trent Gerew’s (AMAT, M.S. AMAT 5th Year) results. He aimed to build and verify a mathematical model using spatial dependence that recreated the known spread of COVID-19 in Chicago with his project, titled “Reaction-Diffusion Spatial Modeling of COVID-19 in Chicago.” Gerew’s goal is to use the model to predict how the disease will spread in the future, test lockdown strategies and travel restrictions, and estimate vaccination thresholds and pockets. It also could be applied to different locations.
“Many models exist already that are used to describe the spread of COVID and other viruses, but few take into account spatial spread,” he says. “They are just a time-only model. There are models that account for diverse populations or demographics. But they become complicated and computationally unfeasible.”
Gerew’s model closely matched the actual spread of COVID-19 on day 11 of the pandemic. His model was off for day 35, but then closely matched actual cases on day 99. A few variables could explain the discrepancy. For example mass gatherings, such as protest marches, were not accounted for. Another possibility is that the reporting of COVID-19 cases and deaths could have been murky. City officials were frantically gathering the data and reporting them sporadically. This isn’t uncommon when a health crisis emerges.
Similarly, Michael Kralis (M.S. AMAT 2nd Year) encountered some data discrepancies as he researched his project “Education Disparities in Chicago Public High Schools.” A graduate of a Chicago Public Schools high school, Kralis developed an algorithm to measure the disparity of CPS students moving on to college after graduation among different demographics.
He cultivated his data from the Illinois State Board of Education and noted the field names differed from year to year, but otherwise the data was reported fairly consistently.
Kralis focused his attention on the college enrollments rates of graduates from public high schools with students from predominantly low-income families. Between 2015 and 2020, the college enrollment rate from these schools’ graduates dropped from 67 percent to 54 percent, while the average of all CPS high schools dropped from 85 percent to 75 percent during the same time period.
“Every time you see a percent of student enrollment positive correlation with a percent of graduates enrolled in college, you almost see the exact opposite in low-income students,” Kralis says.