Long-Term Predictions, Hold the ‘Explosions’
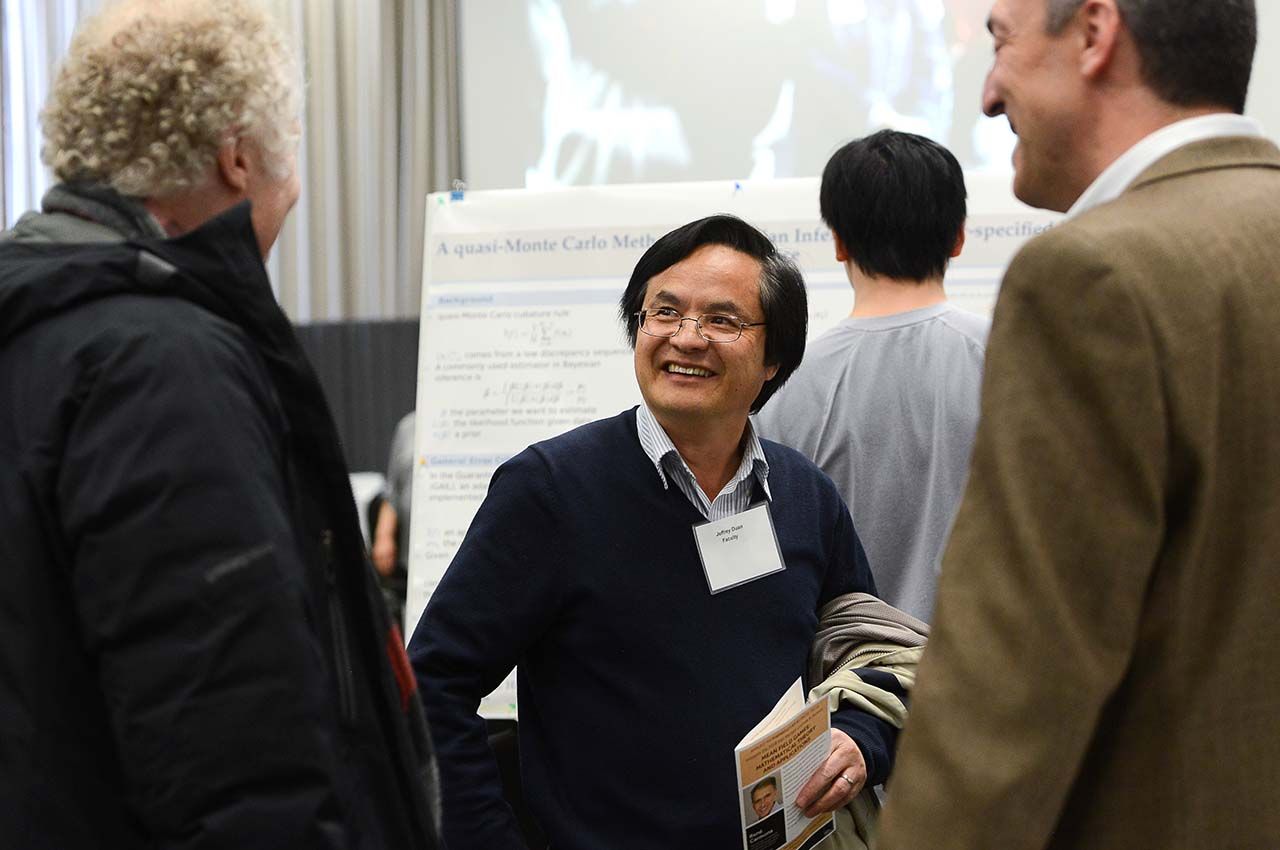
Developing machine learning models that use massive data sets has proved useful in making short-term predictions, but current methods used to make long-term predictions of complex dynamic systems have fallen short.
Researchers from Illinois Institute of Technology have joined a team of scientists to develop stable surrogate models that will be able to make long-term predictions that could benefit research in areas like weather and climate change, or nuclear fusion energy sciences. Jinqiao “Jeffery” Duan, professor of applied mathematics, and Romit Maulik, research assistant professor of applied mathematics and assistant computational scientist at the Mathematics and Computer Science Division at Argonne National Laboratory, will receive $1.2 million of a $3.6 million grant from the United States Department of Energy that was awarded to the research team developing “Inertial Neural Surrogates for Stable Dynamical Prediction.”
“This project will lead us to advances in modeling, analyzing, and predicting dynamical behaviors of complex systems, with new tools in machine learning and data science together with methods in stochastic dynamical systems,” Duan says. “Our research will enable greater confidence in the long term forecasting of crucial applications such as weather and climate dynamics with reduced computational costs.”
“Recently, large quantities of data are being generated by really large simulations and observations from things like satellites and experiments,” Maulik says. “We want to use machine learning to leverage these data sets for surrogate [models] that are as accurate and as stable as possible.”
Maulik explains that the current surrogate models are primarily designed for short-term predictions. However, when the techniques used for short-term predictions are leveraged to make long-term predictions, the models tend to “explode,” or generate results that defy the laws and principles of physics.
“We know that long-term predictions using state-of-the-art methods fail, but this project will study why they do so and design strategies to prevent that,” Maulik says. “We want to leverage our understanding of certain systems to build better methods that do not explode or converge to unphysical solutions.”
The research team will be trying to discover underlying “structure” in data using novel methods, and then use that structure to prevent nonsensical solutions in the long run.
Duan will be leading investigations into the construction of surrogate models for stochastic dynamical systems, leaning on his years of experience in stochastic dynamics research. Maulik brings his expertise in state-of-the-art machine learning, as well as his engagement with Argonne and relevant applications such as weather and climate forecasting.
They join Prasanna Balaprakash and Hong Zhang of Argonne, Joshua Burby and Qi Tang of Los Alamos National Laboratory, and Ioannis Kevrekidis of Johns Hopkins University on the project.
The project is one of five that the DOE is supporting with a $16 million investment in its Data-Intensive Scientific Machine Learning Research and Analysis initiative. Each project focuses on developing reliable and efficient artificial intelligence and machine learning methods for a broad range of scientific needs, including potential applications in areas such as forecasting power grid behavior, predicting extreme weather events, and high-energy physics and cosmology. “Data-Intensive Scientific Machine Learning and Analysis” is sponsored by the Office of Advanced Scientific Computing Research (ASCR) within DOE’s Office of Science.
Disclaimer: "This report was prepared as an account of work sponsored by an agency of the United States Government. Neither the United States Government nor any agency thereof, nor any of their employees, makes any warranty, express or implied, or assumes any legal liability or responsibility for the accuracy, completeness, or usefulness of any information, apparatus, product, or process disclosed, or represents that its use would not infringe privately owned rights. Reference herein to any specific commercial product, process, or service by trade name, trademark, manufacturer, or
otherwise does not necessarily constitute or imply its endorsement, recommendation, or favoring by the United States Government or any agency thereof. The views and opinions of authors expressed herein do not necessarily state or reflect those of the United States Government or any agency thereof."
Photo: Professor of Applied Mathematics Jinqiao “Jeffery” Duan