NSF Grants Illinois Tech Researcher $1 Million for Pioneering Brain Imaging Research
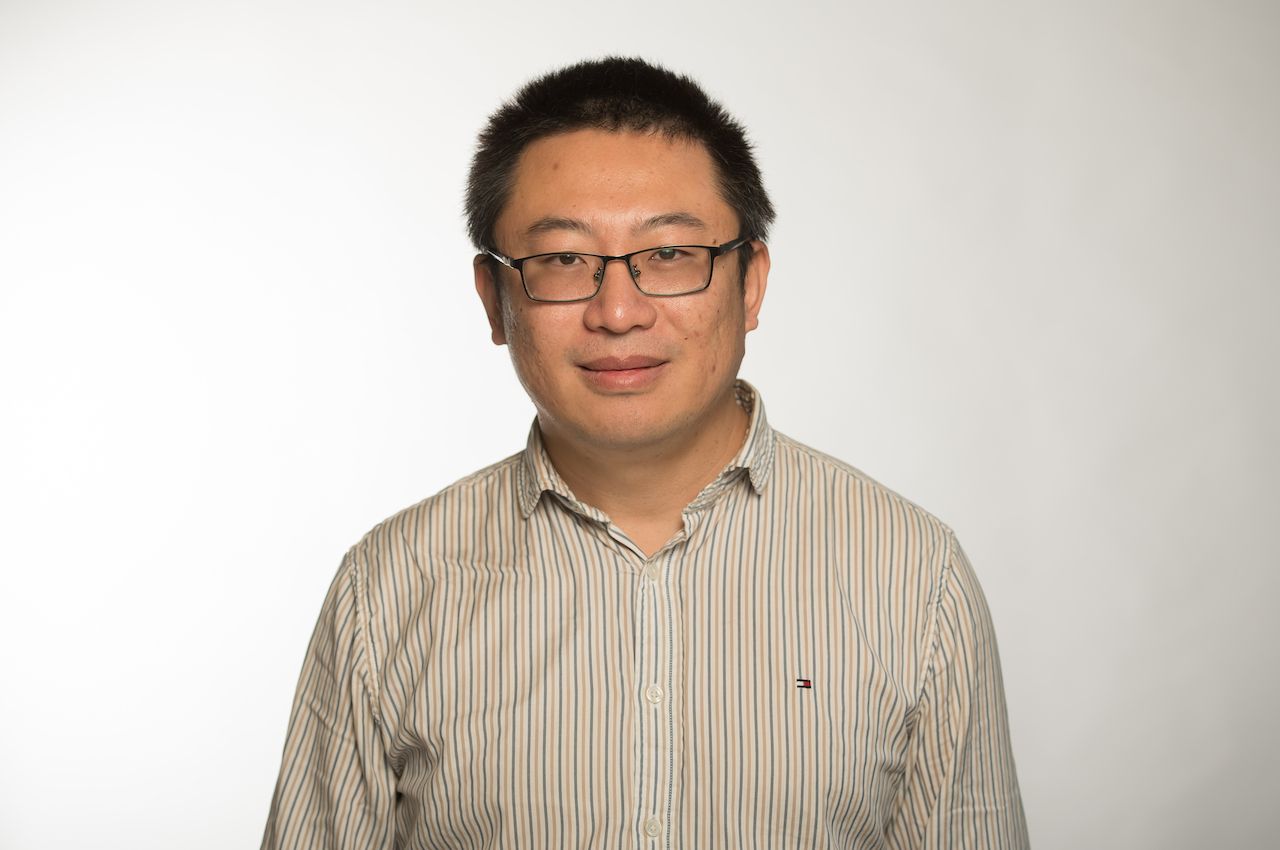
An Illinois Institute of Technology computer science researcher earned a $1 million grant from the National Science Foundation to develop new imaging, machine learning, and computer vision strategies to fully dissect the complex network of neurons in a fruit fly’s entire brain.
Yan Yan, Gladwin Development Chair Assistant Professor of Computer Science, received the award for his project, “A Unified Framework for Unsupervised Sparse-to-Dense Brain Image Generation and Neural Circuit Reconstruction.” Yan has teamed up with Dawen Cai, associate professor of cell and developmental biology and biophysics at University of Michigan, to conduct the research.
“I am a computer scientist with background of computer vision, machine learning, and medical image analysis,” Yan says. “During my postdoc, I already was involved in grant research to perform mapping and imaging of neurons using computer vision techniques.”
The project builds on recent advances in genetic, imaging, and computational methods to develop a unified framework for reliable reconstruction of genetically identified fruit fly brain neurons and their connections at single-neuron resolution from 3D image data.
The technical aims of the project are divided into four main cores. The first will generate novel fruit fly models to acquire Bitbow images with various neural circuit labeling densities. The second will explore a novel unsupervised neuronal structure reconstruction of relatively sparse labeled Bitbow images. Humans then will validate limited numbers of neuron reconstruction gold standards. The third investigates a new densely labeled 3D Bitbow image generation through a multi-source lgmGAN approach. The fourth will construct the neuron via U-shape MLP in densely labeled Bitbow images. Together, these efforts form a novel computational framework to enable accurate, automatic reconstruction of densely labeled neural circuits.
“Our proposed framework is mainly based on the unsupervised approach with limited numbers of neuron reconstruction proofreading, which means we do not require intensive data-labeling in any module of our framework,” Yan says.
Yan says technologies such as expansion microscopy and light-sheet microscopy have enabled high-resolution imaging in large 3D volumes, including the whole fruit fly brain. Several automated solutions to the neuron segmentation problem have been proposed for both light and electron microscopy images. These new approaches should address current computationally intensive and error-prone methods.
“Though the computation and color inconsistency issues are addressed, we can observe that the previous method results in fragmented neurite segmentations,” Yan says. “These fragmented segmentations are flaws in the supervoxelization process, or occlusions by other neurons.”
Current segmentation methods do not provide a tree-like neural tracing structure, which is desirable for many neuroinformatic analyses. However, Yan says the researchers will propose several novel algorithms to address these shortcomings.
“Mapping a whole fly brain is quite a challenging task and we hope the recent developments of AI techniques can assist in achieving this goal,” Yan says.