Quant-ovative: Applied Math Professor Honored for Innovative Approach to Wealth Management
Matthew Dixon and Collaborator Receive Buy-Side Quants of the Year Award from Risk.net
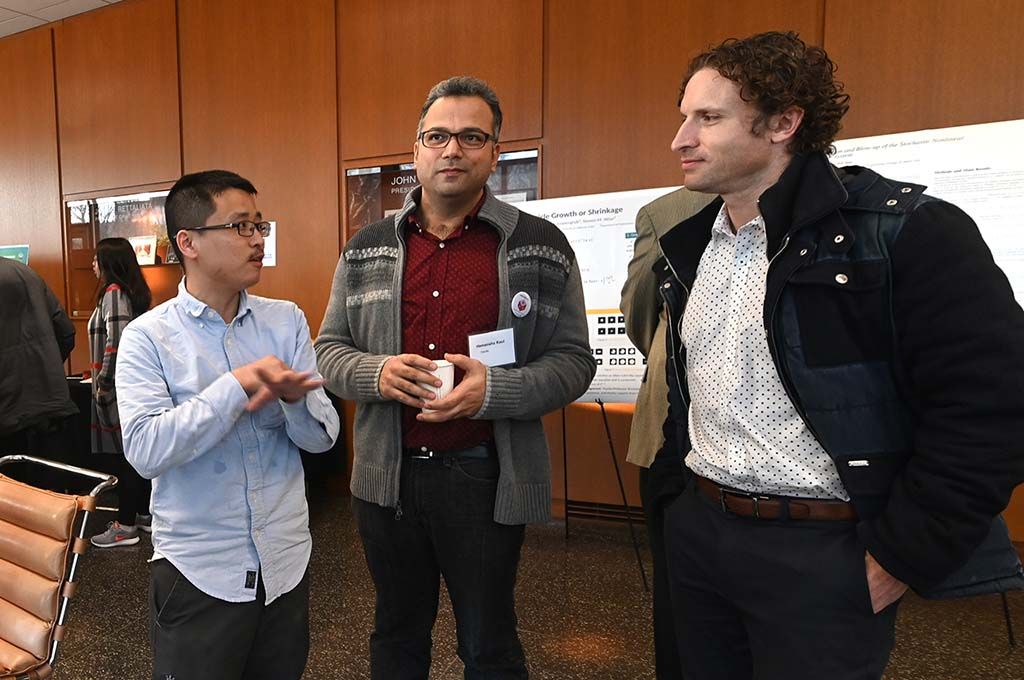
Five years ago Matthew Dixon, assistant professor of applied mathematics at Illinois Institute of Technology, took a gamble and directed his research almost exclusively to the area of machine learning in finance. That decision is already starting to pay off: Dixon was recently named a recipient of the Buy-Side Quant of the Year award by Risk.net.
Dixon, along with Igor Halperin, a vice president at Fidelity Investments’ Artificial Intelligence Asset Management Center of Excellence, earned the award for their joint research using machine learning techniques in optimizing retirement plans and other goal-based wealth management problems.
“Receiving this news is a relief that this initiative paid off and machine learning has become the forefront of quantitative finance,” Dixon says. “Machine learning in finance was not seen as a ‘hot area.’ In fact, it was almost impossible to be recognized without approaching problems from a strictly mathematical and classical approach.”
Machine learning tools and techniques have been used in other fields of finance such as derivatives hedging and risk management, but not in the area of retirement planning—specifically when deciding how to invest and how quickly those savings should be spent.
Dixon and Halperin developed “G-learning,” a probabilistic extension of Q-learning, which is a common reinforcement-learning algorithm. The pair first began collaborating as they were working on a textbook together, Machine Learning in Finance: From Theory to Practice, and continued to work on G-learning after the book was published.
“We both had misgivings about deep Q-learning for many financial applications, and [we] had faced quite a bit of pushback making our views public on this, which motivated us to pursue it further,” Dixon says. “The particular application of optimal consumption is an important one for the wealth management industry.”
Stock prices are very noisy, and fitting deep neural networks to noisy data is like reading too much into the shape of clouds, Dixon says. Dixon and Halperin treat the noise as the weakest link and concentrate on addressing that issue rather than putting emphasis on a deep neural network approximation. Since deep networks aren’t needed for their research, Dixon and Halperin use much simpler approximators, which are much faster to run.
The retirement planning industry has traditionally been a conservative area of finance. Part of the challenge was taking machine learning tools and techniques and translating them into the language and financial modeling framework that allow practitioners to connect the benefits that machine learning brings to wealth management.
The technique also is a seismic shift from the current practice of interviewing fund managers and having them self-report in surveys.
“We, as humans, are subject to bias, and we often act in a way that we believe to be in keeping with our principles, but we sometimes fool ourselves that we are,” Dixon says. “We've also developed a systematic approach which builds on human expertise and skill in managing portfolios and attempts to infer what objective they are actually following when they made past trades.”
Dixon says the research could have far-reaching applications in financial planning—from when to buy a house, budgeting for college tuition, choosing life insurance plans, and tax planning.
“The possibilities are frightening, and there are clear ethical boundaries that must be effective, given access to your health data, your social media data, your credit card data, and more,” Dixon says.
Photo: Assistant Professor of Applied Mathematics Matthew Dixon [right] at Menger Day on March 6, 2020 (Bonnie Robinson)