Tapping Social Media and AI for Faster Disaster Response
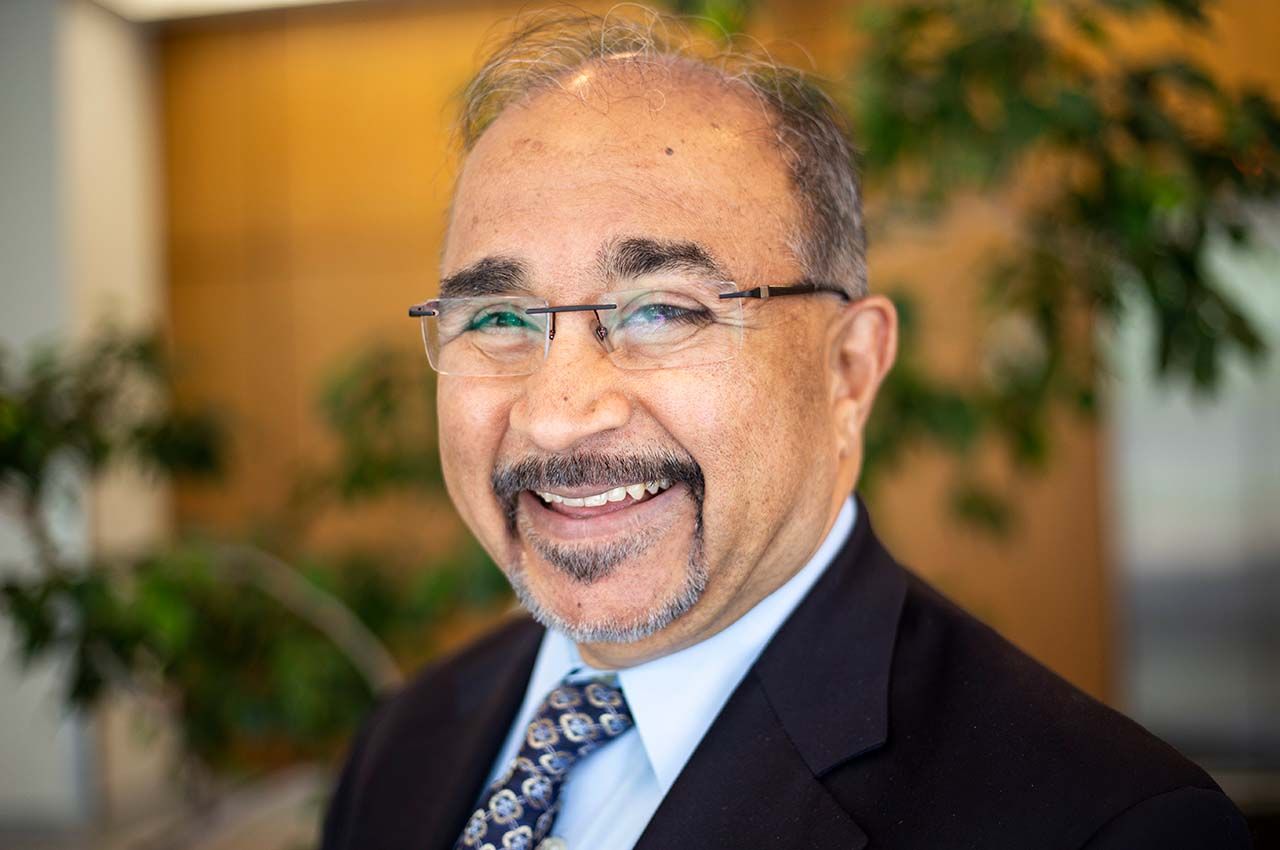
During a natural disaster or crisis, social media platforms are key communication pathways that connect disaster victims with those on the outside—responders, government authorities, and the public—who may be able to provide aid and support.
“Social media allows for instantaneous, direct links to people facing problems during a disaster, and advances in artificial intelligence now make it possible to extract actionable insights from massive amounts of social media data in real time to immediately respond to those people,” says Siva K. Balasubramanian, Harold L. Stuart Endowed Chair in Business at Illinois Institute of Technology’s Stuart School of Business.
In a new study that leverages the latest advances in AI, Balasubramanian and co-researchers Vishwa Vijay Kumar, Avimnyu Sahoo, and Sampson Gholston at the University of Alabama in Huntsville have created a framework for applying AI techniques to optimize social media data during a disaster and mine it for information that can effectively guide response efforts as the disaster is unfolding. Their paper describing the research and results, “Mitigating Healthcare Supply Chain Challenges Under Disaster Conditions: A Holistic AI-based Analysis of Social Media Data,” is forthcoming in the International Journal of Production Research.
For its project, the team used data from X, formerly known as Twitter, from two six-week time periods and two countries during the COVID-19 pandemic: March–April 2020 in the United States when the pandemic broke out and in India during the surge of the delta variant in May–June 2021. At those times, disruptions in health care supply chains were causing severe shortages of essential equipment ranging from face masks and medicines to ventilators for patients in intensive care.
“We conducted a simulation to show how we could use the enhanced AI tools to improve the response to supply chain problems during the pandemic,” Balasubramanian says.
Overall, the researchers analyzed 1.7 million tweets using AI and machine learning in a multi-step process to:
Identify keywords within Twitter posts that would indicate which tweets included information relevant to pandemic supply chain disruptions
Process the tweets for content analysis and modeling
Categorize tweets as “imperative” (actionable pleas for help) and “non-imperative” (providing non-actionable information)
Estimate the geographic location of imperative tweets lacking geo-tag information to facilitate coordination of help operations
In the simulation, Balasubramanian says, “Our goal is to get actionable tweets into the hands of decision-makers when time is of the essence. Once the system is set up, AI is working in the background and much of the process can be automated. An imperative tweet is identified and verified and then AI processes the tweet content to determine action items; that is, what needs to be delivered and where, and when the delivery needs to happen. Then the proper authorities are notified with all of this information, and all of this happens in real time.”
The methods developed in this project can be applied and adapted in a variety of contexts and types of disaster situations, according to Balasubramanian. “The remarkable thing is that AI offers a variety of tools in the toolbox, so the most appropriate tool to solve a specific problem may be deployed; if the problem changes then you use another tool,” he says. “In this research study, we wanted to maximize the societal benefits of using this technology in a disaster context.”