AI Recommends Treatment Options for People with Diabetes to Assist Medical Decision Making
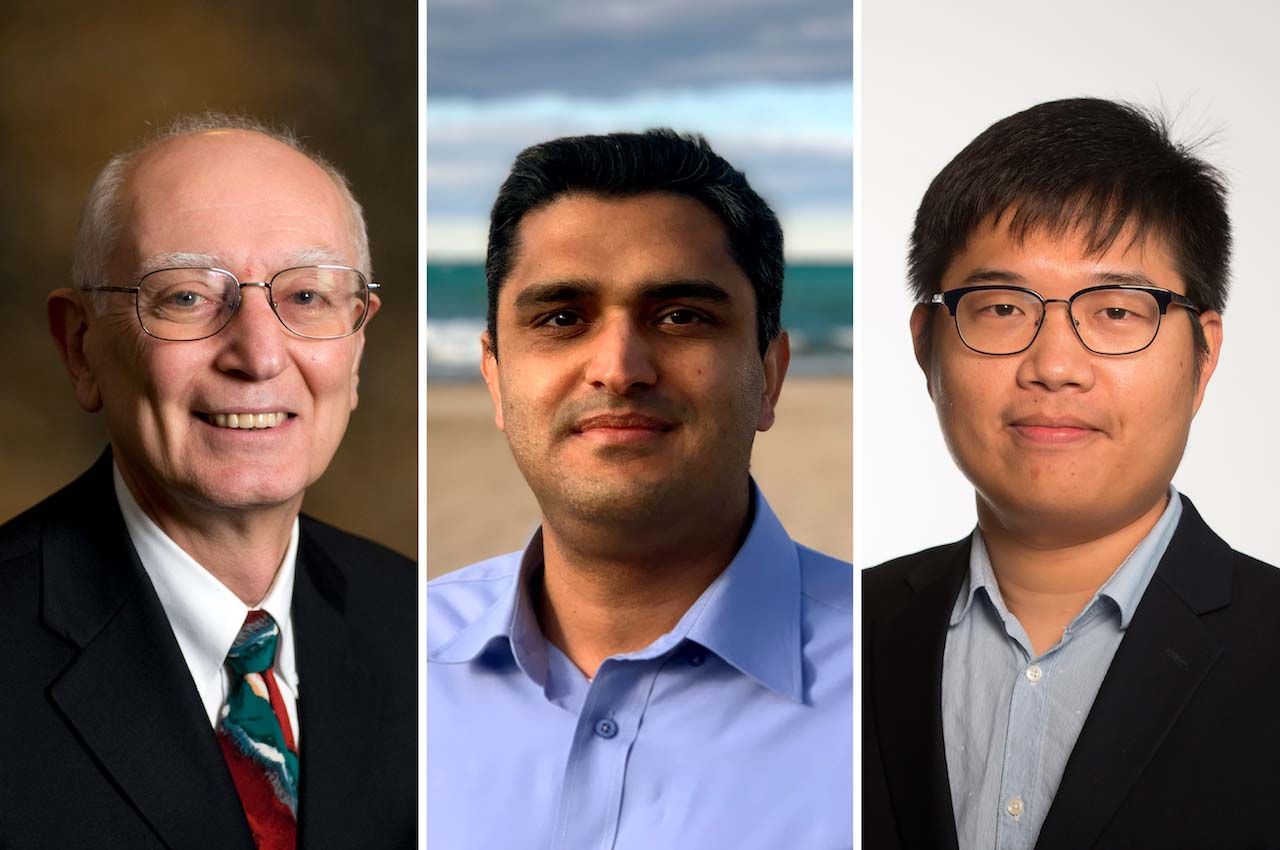
According to the American Diabetes Association, 7.3 million adults in the United States have undiagnosed diabetes. Researchers from Illinois Institute of Technology are part of a research team that is developing new techniques to identify individuals who are unaware that they may be living with the disease and then personalize their treatment.
When identified early, many patients can slow or stop the progression of the disease through medication and lifestyle changes, but when left undiagnosed, diabetes may progress and result in complications such as cardiovascular diseases, loss of touch, blindness, kidney failure, and more.
Director of the Engineering Center for Diabetes Research and Education (ECDRE) and Hyosung S. R. Cho Endowed Chair in Engineering Ali Cinar, Research Assistant Professor Mudassir Rashid, and Gladwin Development Chair Assistant Professor of Computer Science Kai Shu are collaborators on a project that recently received a seed grant from Discovery Partners Institute, which was matched by Illinois Tech, to build techniques and algorithms using artificial intelligence, multivariate statistics, and systems engineering that will help identify undiagnosed diabetes and suggest promising treatments.
Using existing electronic health records provided by collaborators at the University of Illinois Chicago (UIC), the team is building an AI-based model of people with diabetes to improve early diagnosis and selection of the most promising treatment options.
University of Illinois Hospital & Health Sciences System (UI Health) has an initiative involving screening every patient that passes through their emergency department for diabetes. This testing allows for early detection, diagnosis, and treatment of prediabetes and diabetes.
Machine learning with diverse and large-scale electronic health records provides models that can identify the best treatment strategy for each person newly diagnosed with diabetes.
“There is a disparity between different groups in diabetes care, and that starts with the initial detection of diabetes in various populations,” says Cinar. “When people either feel that they’re not too vulnerable or don’t have enough resources, one of the things they cut down are visits to medical facilities, and miss the chances to have their diabetes detected at an early stage.”
When a detection is made, the next step is to identify the most promising personalized treatment options.
“That’s where AI and machine learning come in,” says Rashid. “It is essential to start with electronic health records that include diverse patient populations, and the patient database at UI Health has a good representation of various races and ethnicities.
“We’ll use it to develop unbiased advisory systems to assist medical professionals in selecting the most promising personalized treatments and lifestyle changes for people,” says Cinar.
Currently, doctors tend to offer similar treatment advice to newly diagnosed patients by following guidelines from medical associations and then changing doses or medications depending on how the patient responds, but this work could eliminate that trial-and-error process.
“The simulator we are building will enable the development of virtual twins of patients for rapidly computing the effects of various treatment alternatives and illustrate the best treatment options to medical staff for informed point-of-care decisions,” says Rashid. “It will also educate the patient about the effects of various lifestyle changes.”
“We would be able to show a person with diabetes how walking after meals every day for 10 or 15 minutes and using the specific drug and dose determined by these simulations will help them maintain their blood glucose level within the desired range,” says Cinar. “This will hopefully slow down or stop the progress of the disease, extending their lifespan and improving their quality of life.”
Image: Hyosung S. R. Cho Endowed Chair in Engineering Ali Cinar, Research Assistant Professor Mudassir Rashid, and Gladwin Development Chair Assistant Professor of Computer Science Kai Sh